Transform your business using Artificial Intelligence services.
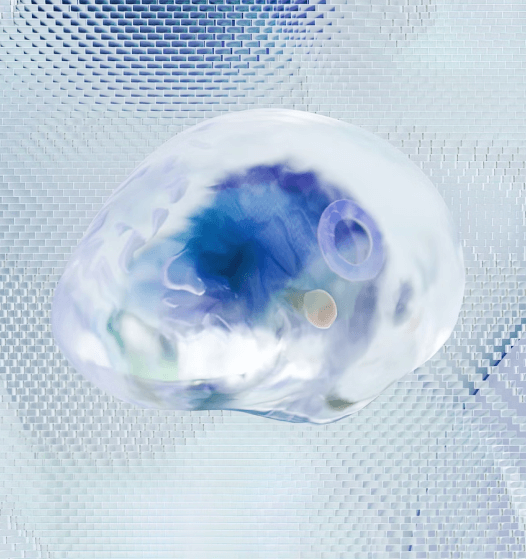
Why you should choose us?
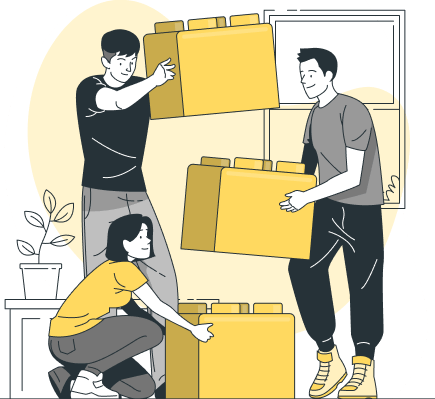
Artificial intelligence Process
Artificial Intelligence (AI) refers to the simulation of human intelligence in machines, enabling them to perform tasks that typically require human cognition. The AI process involves a series of stages from defining a problem, gathering data, preprocessing that data, choosing a suitable algorithm, training a model, evaluating its performance, to deploying it in real-world applications. Throughout these stages, iterative refinements are often required to achieve desired results. The ultimate aim of the AI process is to create models that can make decisions, predict outcomes, or understand patterns in a manner that emulates human-like intelligence, but often at scales or speeds that humans cannot achieve.
Problem Definition
Determine the problem you want the AI to solve. Decide if the problem is best solved through supervised learning, unsupervised learning, reinforcement learning, or another AI method.
Data Collection
- Gather relevant data to train and test the model.
- This might involve collecting new data, using existing datasets, or a combination of both
Data Preprocessing
- Clean the data by handling missing values, outliers, and possible errors.
- Convert textual or categorical data to a numerical format through encoding.
- Normalize or standardize data to bring all features to a similar scale.
- Split data into training, validation, and testing sets.
Model Selection
Choose an appropriate machine learning algorithm or neural network architecture based on the problem type (e.g., regression, classification, clustering).
Model Training
- Feed the training data into the selected algorithm to learn patterns.
- Adjust hyperparameters for optimal performance.
Model Evaluation
- Use the validation set to evaluate the model's performance and tune hyperparameters.
- Apply various metrics (like accuracy, precision, recall, F1 score, etc.) depending on the problem type.
Model Testing
Assess the model's performance using the test dataset to ensure it generalizes well to new, unseen data.
Deployment
- Integrate the trained AI model into the desired application or system.
- This could involve deploying it on a cloud server, integrating it into a software application, embedding it in a device,etc.
Monitoring and Maintenance
- Monitor the model's performance in real-world scenarios.
- Gather feedback and new data to retrain or fine-tune the model as needed.
Iterate
Based on the model's performance, user feedback, and changes in data distribution, regularly go back to previous steps to improve and adapt the model.
Let's talk about your project
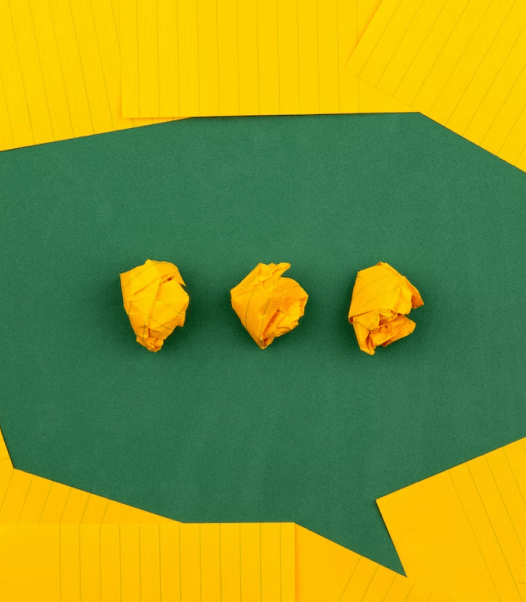